Using AI to make better decisions and optimize business operations

Companies in all industries are under pressure to optimize their operations in a context of high volatility, shortage of labor and improved sustainability. These challenges require typically highly dynamic optimization solutions that are well suited for solving with reinforcement learning (RL). Unlike supervised and unsupervised learning, RL learns to perform tasks as humans often do, through trial and error. RL has matured enough and Apgar is already using it to optimize our customer operations.
Reinforcement Learning (RL) is ready to be used across different industries.
Manufacturing and Industrial RL use cases
RILP can help operational teams manage complex manufacturing processes and industrial systems. It can monitor incoming customer orders in real time to recommend job scheduling actions that meet customer SLAs and minimize raw material losses and human labor. It can be used to create superior software controllers that monitor and recommend parameter values to optimize gas compressor stations or wind turbine operations, for example.
Supply Chain and Other use cases
RL can help organizations monitor supply chains in real time to take the right action as events unfold. A transportation company can optimize travel routes in real time based on truck and labor availability, on changing traffic, and on weather conditions. In the finance and insurance industries RL has also been successful applied in automated trading, portfolio optimization, and in next best action recommender systems.
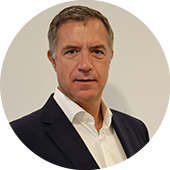
“Reinforcement learning combined with discrete event simulation is a game changer in ensuring the optimal performance of industrial processes and operations when quick decisions have to be taken to answer unexpected events, such as new customer orders and machine failures.”
Concepts and Critical Success Factors.
- Concepts and Critical Success Factors
- Learning Environments and Reward Functions
- Tunning and Computing Resources
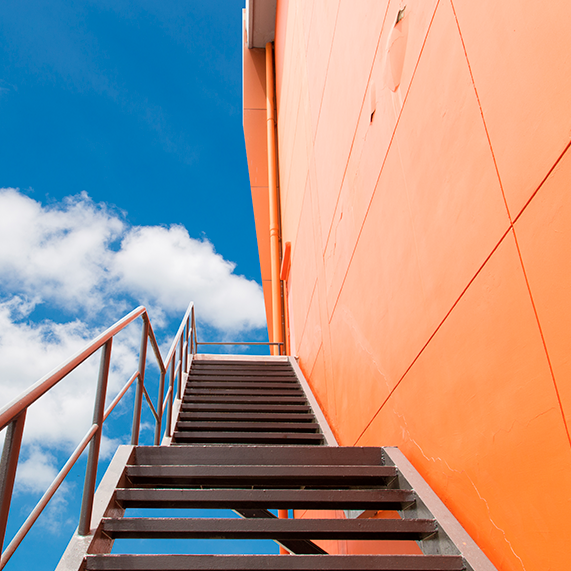
How Reinforcement Learning Works
A RL agent learns through trial and error. Simply put, the RL agent observes the state of a process or system (often a simulation or digital twin), performs actions on it, and receives rewards (positive or negative) for each action. The learning process consists in finding the sequence of actions that maximizes the cumulative rewards it receives during a specific time-period or to accomplish a specific task.