Enable Operational Excellence with ApgarIA

Experience unparalleled operational efficiency with ApgarIA, our Intelligent Agents for Operations. Our cutting-edge technology, powered by advanced artificial intelligence, is designed to optimize industrial operations and improve productivity. We leverage advanced analytics and AI to analyze complex data, optimize production and deliver actionable insights to drive operational excellence and give your business a competitive edge.
AI-Powered Optimization for Smarter, Cost-Effective Operations.
- Increase production efficiency and lower operational costs ApgarIA’s capacity planning and production scheduling optimization solution uses AI to generate detailed production schedules and capacity analyses in seconds. It revolutionizes planning agility by reducing changeover and stock costs and eliminating dependence on outdated tools and human heuristics.
- Optimize Inventory and procurement costs: ApgarIA’s demand forecasting solution leverages advanced analytics and machine learning to accurately forecast demand and optimize sourcing. This empowers businesses to meet customer needs while maintaining cost-effective operations. Stay ahead of the curve with precise demand forecasting and optimized procurement.
- Improved operational efficiency and reduced downtime: ApgarIA’s predictive analytics for IoT data uses specialized machine learning models to identify abnormal data patterns and time-related relationships, generating intelligent alerts and advanced predictive operations. From predicting the remaining useful life of equipment in a factory to determining the ideal harvesting date in a farm, we’ve got you covered.
- Smarter and faster operational decisions: ApgarIA includes an AI Assistant similar to ChatGPT but purpose-built for business operations. Imagine having a virtual operations expert at your fingertips. This AI Assistant is trained with your documents and enriched with your industry and organization-specific business context, ready to assist any employee with any query or recommend a particular course of action.
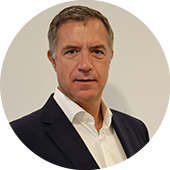
"ApgarIA's solutions are revolutionizing production and inventory management, optimizing costs and boosting the efficiency of our manufacturing customers. By harnessing the power of advanced analytics and AI, we're empowering industrial companies to respond proactively to market changes, reduce waste, and drive profitability, all while enhancing customer satisfaction and loyalty."
Our solutions.
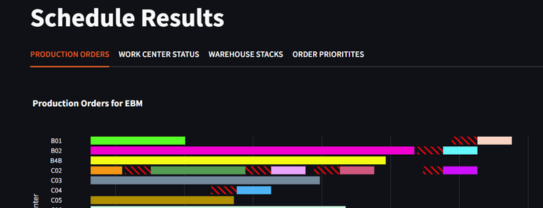
Production Scheduling Optimization - Apply AI to generate optimal production schedules in real time.
AI-Optimized Production Scheduling & Capacity Studies
Faster, Smarter, More Efficient Planning : Planning inefficiencies cost time and money. ApgarIA leverages AI and constraint programming to:
Automate and Optimize Schedules: Reduce idle time and increase production output.
Minimize Changeovers: Cut unnecessary production changeovers by up to 30%.
Reduce Warehouse Stock: Maintain optimal inventory levels with smarter planning.
Optimize Long Term Supply Planning: Reduce the long-term resources requirements with long term production planning optimization.
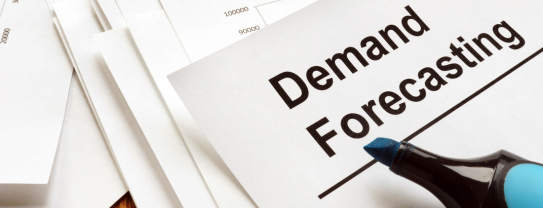
AI-powered Demand Forecasting
Turn Forecasting into a Competitive Advantage
Traditional forecasting methods often rely on historical trends, missing key external and operational factors. ApgarIA’s AI-driven forecasting brings:
Advanced Machine Learning Models: Pre-built statistical models, advanced machine learning models, and more.
Scenario Analysis: Create and compare different forecasting scenarios and generate supply demand plans.
Accuracy Visibility and Improvement: Evaluate forecast performance and identify improvement opportunities.
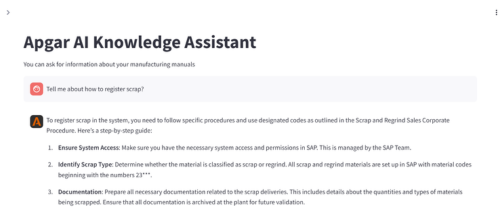
AI Assistant for Operations - An AI Assistant like ChatGPT but purpose-built and customizable for business operations.
Empower any user to become an Operations Expert
Technicians and operators waste valuable time manually searching for critical operational information, slowing operations and often leading to errors. ApgarIA’s AI Operations Assistant eliminates these inefficiencies by providing:
Instant Answers: Retrieve key insights from internal and external data sources.
Context-Specific Responses: AI trained on your unique operations documents and data.
Increased Efficiency: Reduce manual research time for content and data, improve decision-making.
Our ApgarIA Assets.
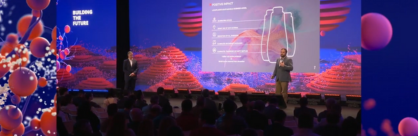
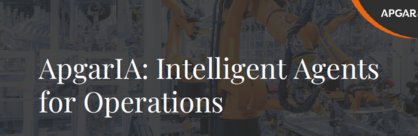
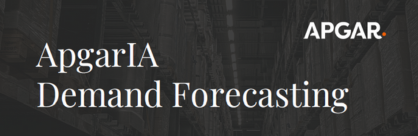
Questions about our offering or solution? Remarks to share?
Mário is available to advise and support you across your AI projects.
Mário DUARTE
Business Director, Head of AI & Advanced Analytics
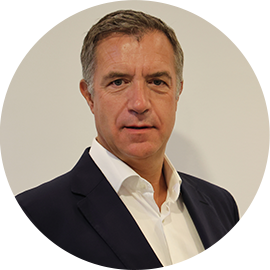